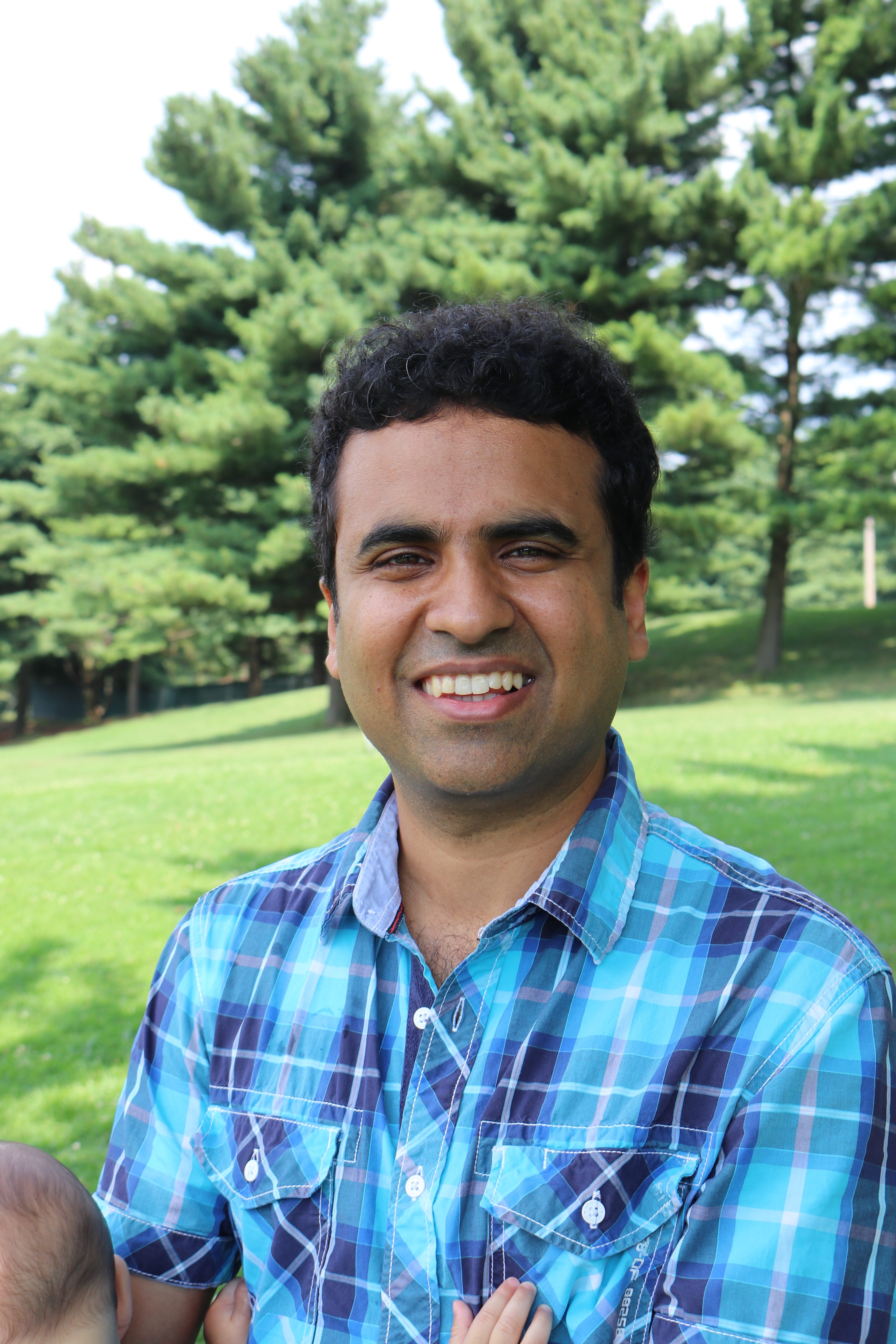
Pulkit Grover
Professor
Electrical & Computer Engineering;
Neuroscience Institute;
Biomedical Engineering (by courtesy);
and
the Center for Neural Basis of Cognition
B-202 Hamerschlag Hall
Carnegie Mellon University
Ph: (412) 268-3644
pgrover at andrew dot cmu dot edu
Image credit: Kris Woyach
Broadly, I am interested in an understanding of information that goes beyond just communication, and particularly intersects with neuroscience and neuroengineering, as well as modern AI systems. We use a mix of thought and laboratory experiments, spanning examination of fundamental limits all the way to experiments (with animals and humans) to test and obtain new insights at these intersections. The "For All Lab" I lead focuses on engineering principles of devices and systems that are accessible by all (minimally/non-invasive) and are unbiased (e.g. towards hair-type and skin-color). Current topics of interest include flow of information in neural systems and neural interfaces (and use of this understanding to design radically new neural interfaces to diagnose, manage, and treat disorders); and fundamental and practical understanding of networks that process information or attain inferences. Find a short bio here.
Postdoc (2011-12), Electrical Engineering, Stanford.
PhD, EECS, UC Berkeley, Dec 2010.
B. Tech, M.Tech, IIT Kanpur ('03, '05)
Schooling: Vidyashram, Jaipur
You can find my CV here.
Accessible, bias-free non-invasive neural sensing and diagnosis: theory, algorithms, implementation, and clinical translation
We are seeking a fundamental approach, guided by signal processing and information theory, to understand information-use in wearable and implantable biosensing systems.
New ways of diagnosing neural disorders (supported by the Chuck Noll Foundation for Brain Injury Research, NSF, and CMLH): Our efforts have been focused on stroke and traumatic brain injuries. Collaborating with clinicians at University of Pittsburgh, MGH, and U Cincinnati, led by Alireza Chaman Zar (who also created the above gif), we obtained the first non-invasive detection of cortical spreading depolarizations (CSDs), also called Brain Tsunamis, in severe traumatic brain injury patients, and the first algorithms for localizing neural silences. Recent work extends these solutions to improved stroke diagnosis in the “hyper-acute” window (led by Yuxin Guo).
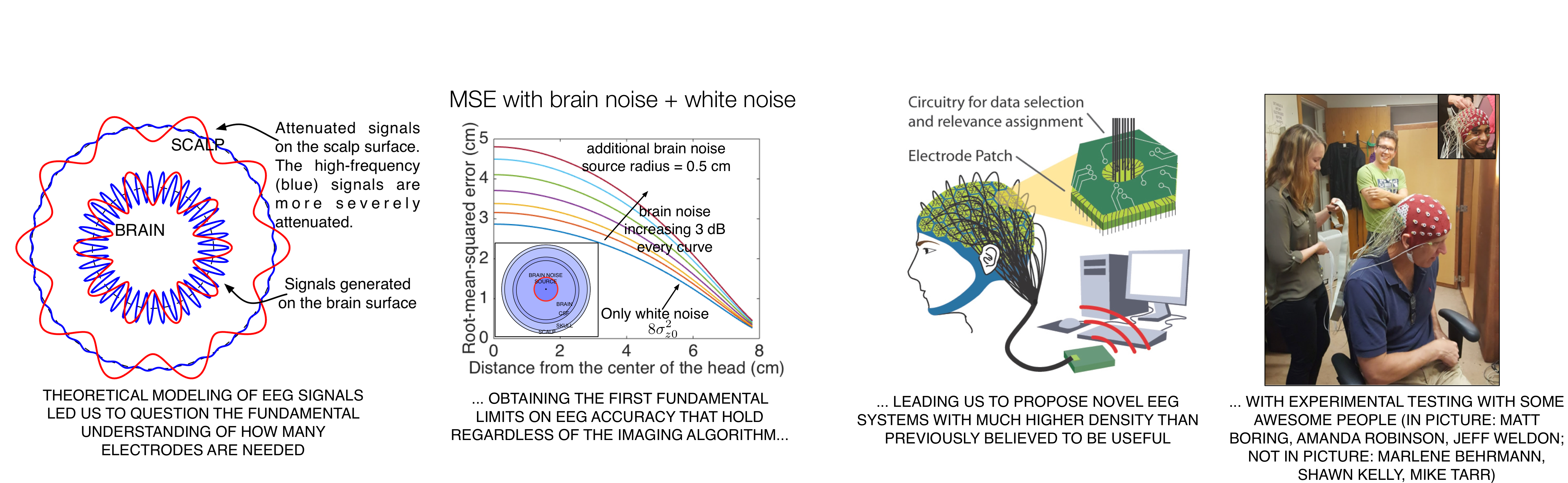
Fundamental limits: For noninvasive (EEG) brain sensing modality, our work [Proc IEEE'17, ISIT'17] (support: SRC SONIC, CMU BrainHUB, and NSF WiFiUS) makes a systematic case that the current theoretical understanding severely underestimates EEG's spatial resolution. In essence, it relies on a spatial Nyquist rate estimation when, really, Nyquist rate (as estimated) has nothing to do with how much information you can infer about the brain activity from the scalp. Recently, we have been able to obtain the first experimental validations for these conclusions (with Marlene Behrmann's lab, led by Amanda Robinson and Praveen Venkatesh; see [Robinson et al.'18]), and are working with clinicians on using this high resolution for improved diagnoses of neural disorders. This has led us to instrument of some of the highest density EEG systems to exist (for their coverage). Working with instrumentation engineers (led by Ashwati Krishnan, Ritesh Kumar) on overcoming novel challenges and difficulties in instrumenting and installing such high density systems. I am personally very interested in seeing these systems to their end goal: improvement in clinical and neuroscientific inferences.
Bias in EEG systems: Our work [Etienne et al., EMBC'20], supported initially by the Chuck Noll Foundation, reported, for the first time, that EEG signal quality is worse for state-of-the-art EEG systems when used with coarse and curly hair common in individuals of African descent. The first solutions to this problem, developed by Arnelle Etienne, have been commercialized by a startup (Precision Neuroscopics, Inc.). The work has received a lot of attention, which is helping educate clinical technologists and healthcare systems about the problem with existing EEG systems, and helping existing companies work on improving their solutions. The work is now supported by an NIH STTR award, Pittsburgh Innovation Challenge, and a Block Center Award. We are aiming to translate these solutions to clinics in Africa through an Afretec award.
Information flow definition and inference: A fundamental question is: how well can we infer information flow directions in the brain? Our information-theoretic counterexamples [Allerton'15c] (inspired by the celebrated work of Schalkwijk & Kailath) show that Granger causality and directed information-- used widely to infer these directions -- can provide the wrong answer even when there is no missing node! These information flow-directions are important to infer in order to understand minimialist approaches to treatments of neural disorders through e.g., stimulation, drug delivery, or resection/ablation. Worse, the obtained insights are, well, not that great. E.g., you get a sense of direction of (some vaguely defined) influence, but nothing more. Recently, [IT Trans'20], led by Praveen Venkatesh and Sanghamitra Dutta, we provided the first formal definition of information flow in computational systems that satisfies intuitive properties and also provides finer-grained information than Granger causality-type techniques aim to provide. Ongoing work is testing this on simulated and real neural data.
Salient references (full list here)
[IT Trans'20] P Venkatesh, S Dutta, P Grover. Information flow in computational systems. IEEE Transactions on Information Theory 66 (9), 5456-5491, 2020.
[Nat. Comm. Bio'21] A Chamanzar, M Behrmann, P Grover. Neural silences can be localized rapidly using noninvasive scalp EEG. Nature Communications Biology, 2021.
[EMBC'20] A Etienne, T Laroia, H Weigle, A Afelin, ... , A Krishnan, P Grover. Novel Electrodes for Reliable EEG Recordings on Coarse and Curly Hair 42nd Annual International Conference of the IEEE Engineering in Medicine and Biology, 2020.
[ISIT '17a] Praveen Venkatesh and Pulkit Grover. Lower Bounds on the Minimax Risk for the Source Localization Problem IEEE International Symposium on Information Theory (ISIT). Aachen, Germany, July 2017.
[Proc. IEEE '17] Pulkit Grover and Praveen Venkatesh. An information-theoretic view of EEG sensing, Proceedings of the IEEE, special issue on Science of Information (edited by Tsachy Weissman, Ananth Grama, Tom Courtade).
[Allerton '15c] Praveen Venkatesh and Pulkit Grover. Is the direction of greater Granger causal influence same as the direction of information flow? Annual Allerton Conference on Communication, Control, and Computing, 2015.
[Nat. Sci. Rep '18] Amanda K. Robinson, Praveen Venkatesh, Matthew J. Boring, Michael J. Tarr, Pulkit Grover and Marlene Behrmann. Very high density EEG elucidates spatiotemporal aspects of early visual processing. Nature Scientific Reports, Jan 2018.
Controlling the neural chaos using neurostimulation
We are investigating strategies that harness the nonlinear dynamics of neurons to attain localized noninvasive current stimulation (support: DARPA N3). This is an exciting collaboration with the Weber, Chamanzar, and Fedder Labs at CMU, where, led by Dr. Mats Forssell, Dr. Vishal Jain, and Chaitanya Goswami, we have developed novel machine-learning and optimization algorithms, tested them rigorously in animal studies, and area translating them into the clinic.
We developed theoretical and optimization techniques to optimize fields created by injected currents, yielding focal responses, and tested these in non-invasive studies in animals (rodents and NHPs) and healthy humans. We are seeking to translate these to treatment of chronic pain, stroke, and other diseases and conditions.
Separately, we are developing AI techniques for neurostimulation. A key goal is to optimize both spatial pattern of current injection [EMBC'21] and temporal waveform design [TBME'23]. This creates an extremely large search-space, in which Chaitanya Goswami's work seeks to perform a systematic search, enabling finding optimized stimulation parameters.
Salient references (full list here)
[IEEE TBME'19] J Cao, P Grover. STIMULUS: Noninvasive dynamic patterns of neurostimulation using spatio-temporal interference. IEEE Transactions on Biomedical Engineering 67 (3), 726-737, 2019.
[JNE'21] M Forssell*, C Goswami* (co-first authors), A Krishnan, M Chamanzar, P Grover. Effect of skull thickness and conductivity on current propagation for noninvasively injected currents. Journal of Neural Engineering 18 (4), 2021.
[EMBC'21]Goswami, Chaitanya, and Pulkit Grover. "HingePlace: Focused transcranial electrical current stimulation that allows subthreshold fields outside the stimulation target." 43rd Annual International Conference of the IEEE Engineering in Medicine & Biology Society (EMBC), 2021.
[TBME'23] Goswami, Chaitanya, and Pulkit Grover. "PATHFINDER: Designing Stimulus for Neuromodulation through Data-driven Inverse Estimation of Non-linear Functions." IEEE Transactions on Biomedical Engineering (2023).
[AS'23] Jain, V.*, Forssell, M.* (co-first authors), Tansel, D. Z., Goswami, C., Fedder, G. K., Grover, P., & Chamanzar, M. (2023). Focused Epicranial Brain Stimulation by Spatial Sculpting of Pulsed Electric Fields Using High Density Electrode Arrays. Advanced Science, 2023.
Coded computation for resilient computing with unreliable elements: fundamental limits and practical strategies
Modern distributed computing systems, from nanoscale circuits to large supercomputers, are all prone to faults, errors, and delays in computing. Our approach is to merge computation and error-correction coding into “coded computation” that uses close to optimal redundancy in making the system robust to faults/errors/delays. This is attained through obtaining fundamental limits as well as novel coded computation strategies, and comparing them. Our focus is on examining applications of modern interest, such as scientific computing and machine learning, and in identifying building blocks of these computations towards making them resilient.
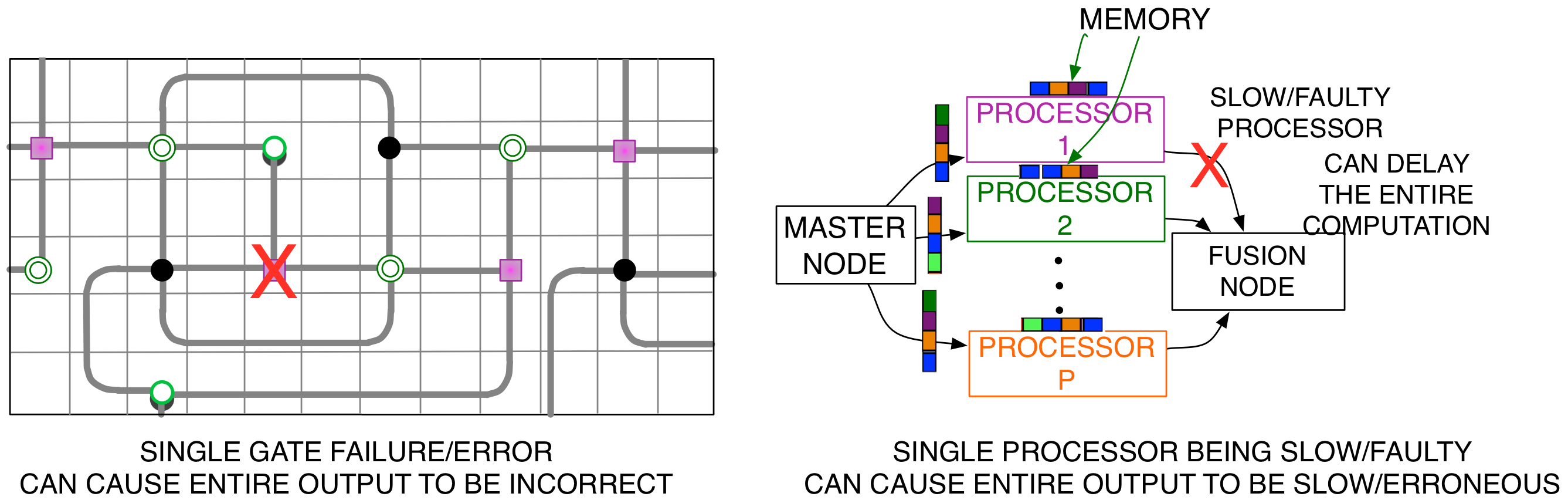
This raises new coding theory problems, e.g. low communication-complexity ``Short-Dot'' codes for matrix multiplication. At ISIT'17, I will jointly present a tutorial on this exciting area, discussing fundamental limits and strategies for combating errors (with Viveck Cadambe, Penn State).
What is fundamentally new in this area that goes beyond classical information and coding theory? I have observed two important aspects:
- Deep understanding of encoding and decoding costs is required: our fundamental limits on reliability/precision and energy tradeoffs [ISIT'14] conclusively show that viewing noisy circuits merely as noisy ``channels'' and computing Shannon capacities can provide misleading insights because this view ignores encoding/decoding computations.
- Errors accumulate, as recent works on ``strong'' data-processing inequality show. In fact, for quantized distributed computing, our new techniques [TIT'17a] reveal that the classical cut-set bounds are loose by an arbitrarily large factor because they do not capture this accumulation.
These fundamental results provide insights that we used to arrive at efficient and robust strategies: careful embedding of error-correction at intermediate stages of computation can be used to control error-propagation. Our results [TIT'17b] are the first strategies that, despite all gates being noisy, are able to compute linear transforms “reliably”. For pursuing this direction, we enthusiastically joined the SRC SONIC center enabling tech-transfer to industry, and also recently (2017) received an NSF grant.
Salient references (full list here)
[TIT '17b] Yaoqing Yang, Pulkit Grover, and Soummya Kar. Computing Linear Transformations with Unreliable Components, IEEE Transactions on Information Theory, Vol 63, No. 6, June 2017.
[TIT '17a] Yaoqing Yang, Pulkit Grover, and Soummya Kar. Rate Distortion for Lossy In-network Function Computation: Information Dissipation and Sequential Reverse Water-Filling, IEEE Transactions on Information Theory, to appear, 2017.
[NIPS '16] Sanghamitra Dutta,Viveck Cadambe, and Pulkit Grover.“Short-Dot”: Computing Large Linear Transforms Distributedly Using Coded Short Dot Products. Advances on Neural Information Processing Systems (NIPS).
[ISIT '17] Sanghamitra Dutta,Viveck Cadambe, and Pulkit Grover. Coded convolution can provide arbitrarily large gains in successfully computing before a deadline. IEEE International Symposium on Information Theory (ISIT). Aachen, Germany, July 2017.
Slides for my ISIT'17 tutorial on Coded Computation (with Viveck Cadambe): here and here. Big thanks to Yaoqing Yang, Sanghamitra Dutta, and Haewon Jeong for their help!
Understanding Information and Computational Complexity:
Theoretical underpinnings and practical designs of “green” radios;
The goal is to develop a fundamental and relevant understanding of complexity of coding and communication techniques. Intellectually, complexity of coding is a missing piece that limits our larger understanding of “information”. Practically, today's short-distance communication systems require processing power that dominates transmit power, sometimes by orders of magnitude.
Theory: Developing a fundamental understanding of complexity of coding has proven extremely difficult: fundamental limits on complexity of problems are in general hard to obtain. My work establishes the first such fundamental limits on encoding and decoding power. The novelty that allows me to do so is to use models based on communication complexity, rather than Turing complexity (number of operations). Simultaneously, for today's circuits, communication complexity turns out to be more closely related to power consumption than number of operations.
Practice: Taking these results towards practice, I use these bounds as guiding principles behind code designs. Not only does this lead to new code-design problems (see [Globecom '12]), we also show that this approach of jointly designing code/encoder/decoder triples (rather than designing them in isolation) has the potential to significantly reduce power consumed in short-distance communication systems.
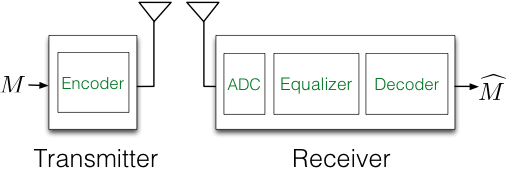
Main contributions
Theory: Our main contribution is to provide fundamental limits on total energy/power required for communication. These limits offer a different picture when compared with the traditional (transmit-power-only) Shannon-limit.
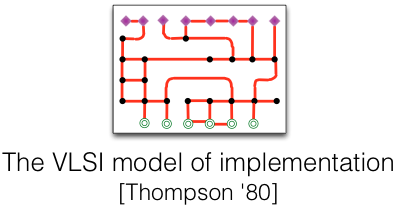
Conclusions derived from our “Node Model” and “Wire Model” are as follows:
- [Node model] There is a fundamental tradeoff between transmit and encoding/decoding power. When computational nodes dominate processing power, to minimize total power, one must fundamentally stay away from capacity.
- [Node model] Capacity-approaching LDPC codes optimize over transmit power, but require large decoding power. Regular LDPC codes are order-optimal in the Node Model.
- [Wire/Info-friction model] When wires dominate the circuit power consumption, the total power diverges to infinity significantly faster than that for the node model. Further, the optimal choice of transmit power also increases unboundedly as the error probability is lowered.
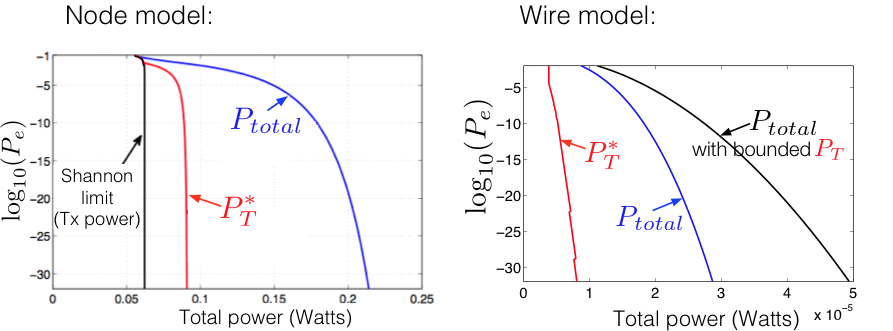
Practice: What are the best codes now? A joint theory-practice approach based on modeling of circuit-power using rigorous circuit simulation shows that the code construction and the complexity of decoding algorithm needs to increase as the target error probability is lowered or as the communication distance is increased.
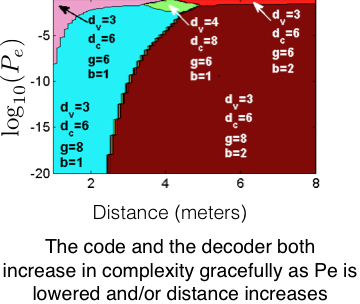
Our results have the potential to drastically reduce power consumed in short-distance wireless (e.g. 60 GHz band) and wired (e.g. multi-Gbps communication in data-centers) communication.
Salient references (full list here)
Theory:
[TIT'15a] Pulkit Grover, “Information-Friction” and its Implications on Minimum Energy Required for Communication, IEEE Trans Information Theory, Feb. 2015, vol 61, issue 2, 895-907 [PDF]
[JSAC '11] Pulkit Grover, Kristen Ann Woyach and Anant Sahai, Towards a communication-theoretic understanding of system-level power consumption, IEEE Journal of Selected Areas in Communication (JSAC) Special Issue on Energy-Efficient Wireless Communications. [PDF][MATLAB Code]
[ISIT'17c] Haewon Jeong, Christopher Blake, and Pulkit Grover. Energy-Adaptive Polar Codes: Trading Off Reliability and Decoding Energy with Adaptive Polar Coding Circuits. In: IEEE International Symposium on Information Theory (ISIT). Aachen, Germany, July 2017.
[ISIT '12] Pulkit Grover, Andrea Goldsmith and Anant Sahai. Fundamental limits on complexity and power consumption in coded communication. Proceedings of the IEEE International Symposium on Information Theory (ISIT), 2012. [PDF]
[ITW '07] Pulkit Grover, Bounds on the Tradeoff between decoding complexity and rate for codes on graphs, Proceedings of the IEEE Information Theory Workshop (ITW) 2007, Lake Tahoe, CA, 2007. [PDF]
[ISIT '14] Pulkit Grover. Is “Shannon-capacity of noisy computing” zero? Proc. IEEE ISIT'14.
Experiments:
[JSAC '16a] Karthik Ganesan, Pulkit Grover, Jan Rabaey, and Andrea Goldsmith. Towards Approaching Total-Power-Capacity: Transmit and Decoding Power Minimization for LDPC Codes, IEEE Journal on Selected Areas in Communication (JSAC), 2016. Special issue on capacity-approaching codes.
[Globecom '12] Karthik Ganesan, Yang Wen, Pulkit Grover, Andrea Goldsmith and Jan Rabaey, Mixing theory and experiments to choose the “greenest” code-decoder pair, Globecom 2012.
“Understanding Information and its Use:
Control and communication in cyber-physical systems”
The goal: How do we connect the “cyber” -- the computational/ communicating/ controlling agents -- with the “physical” -- the world around us? My dissertation lays down the foundations of a theory to help answer this question.
The question: Let us consider two control agents that are connected using a noisy communication link. How should they share their workload? I was surprised to find that we do not know the answer! This is because most of the problem formulations and solution techniques in this field are inspired from those in modern communication theory, and simplify the control agents into “observers” or “controllers.”
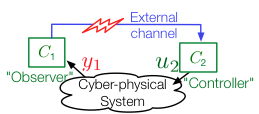
The “observers” cannot act on the system, and therefore they communicate their observations to the “controllers.” The “controllers” cannot observe the state directly, and thus rely on the signals sent by the “observers” to decide on their actions. In a realistic control system, these simplified control agents may be extremely limiting. However, analytically, they have been simpler to understand because they disallow implicit communication through the system from the observer to the controller.
A theory of implicit communication:
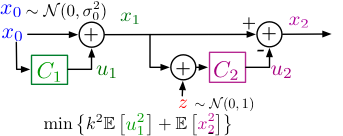
The crux of this issue is captured in a deceptively simple problem called the Witsenhausen counterexample, which has been open despite more than 40 years of research effort. In my dissertation, I provide the first provably approximately-optimal solution to the Witsenhausen counterexample by understanding the potential of implicit communication between the controllers. These approximately-optimal solutions were developed in the following sequence of results. In [CDC '08, IJSCC '10, ITW '10], we introduce a vector version of Witsenhausen's counterexample, and obtain approximately-optimal solutions for an asymptotically infinite- length version of the problem. In [ConCom '09, CDC '09, TAC '10 Sub.], we pull the results back to finite-lengths, including the original (scalar) counterexample, using techniques from large-deviation theory.
Building a communication theory for decentralized control: In my dissertation, I argue that the Witsenhausen counterexample has been a major bottleneck in understanding the interplay of control and communication. Because the counterexample was viewed as essentially unsolvable, it forced problem formulations into corners where the obtained engineering insights could only be very limited. Our approach of using semi-deterministic models to obtain approximately-optimal strategies can be extended to understand toy problems of control under commu- nication constraints which could not have been understood earlier. For instance, what happens when controllers can communicate implicitly as well as explicitly [Allerton '10]? How should one communicate implicitly in a dynamic setting with feedback [Ph.D. thesis]? Various extensions of the counterexample appear in [Allerton '09, ISIT '10, CDC '10].
Salient references (full list here)
[Ph.D. thesis] Pulkit Grover. Actions can speak more clearly than words. Ph.D. thesis, UC Berkeley, Dec. 2010
[TAC '13] Pulkit Grover, Se Yong Park, and Anant Sahai, The finite-dimensional Witsenhausen counterexample. IEEE Transactions on Automatic Control, Sep 2013. [PDF] [Talk Handout] [Talk Slides][MATLAB code]
[TIT '15b] Pulkit Grover, Aaron B. Wagner and Anant Sahai, Information Embedding and the Triple Role of Control. IEEE Transactions on Information Theory, 2015. [Short description] [PDF] [MATLAB code]
[IJSCC '10] Pulkit Grover and Anant Sahai, Vector Witsenhausen Counterexample as Assisted Interference Suppression. Special Issue on "Information Processing and Decision Making in Distributed Control Systems" of the International Journal of Systems, Control and Communications (IJSCC), 2010.
[ISIT '10] Pulkit Grover and Anant Sahai, Distributed signal cancelation inspired by Witsenhausen's counterexample. IEEE International Symposium on Information Theory (ISIT) 2010. [expanded PDF] [Proceedings version]
[CDC '08] Pulkit Grover and Anant Sahai, A vector version of Witsenhausen's counterexample : Towards convergence of control, communication and computation. Proceedings of the IEEE Conference on Decision and Control in Cancun, Mexico, 2008. [PDF] [Handout] [Slides]
People who make our For All Lab.
Research Scientists and Postdoctoral researchers
- Jasmine Kwasa
- Mats Forssell (jointly with Maysam Chamanzar)
- Vishal Jain (jointly with Maysam Chamanzar)
Students and RAs
Ph.D. students
- Neil Ashim Mehta (ECE; jointly with Patricia Figueiredo, ISR, IST, Lisbon)
- Amanda Merkley (ECE)
- Yuhyun Lee (BME)
- Yuxin Guo (PNC)
- Diogo Mendes Cardoso (ECE; jointly with Patricia Figueiredo, ISR, IST, Lisbon)
- Rabira Tusi (MSTP/PNC)
- Jeehyun Kim (BME)
- Kora Hughes (ECE)
B.S., M.S. and post-bac RAs
- Evangeline Mensah-Agyekum
- Alonso Buitano Tang
Lab alums
PhD Student Alums
- Yaoqing (Jordan) Yang (now an Assistant Professor at Dartmouth College; previously a postdoc at UC Berkeley)
- Haewon Jeong (now an Assistant Professor at UCSB; previously postdoc at Harvard)
- Praveen Venkatesh (now the Shanahan Foundation Fellow postdoc at Allen Institute of Brain Science)
- Sanghamitra Dutta (now an Assistant Professor at University of Maryland College Park; previously a Researcher at JP Morgan)
- Jiaming Cao (now a postdoc at the University of Birmingham)
- Alireza Chamanzar (now an Assistant Professor at University of Pittsburgh)
- Chaitanya Goswami (now a postdoc at University of Pittsburgh)
Postdoc Alums
- Sarah Haigh (joint with Marlene Behrmann; now faculty at UNR)
- Amanda Robinson (joint with Marlene Behrmann; now a Research Fellow at Queensland Brain Institute)
- Ashwati Krishnan (now at StimScience, a neurotech startup)
MS and Undergraduate Student Alums (too many! If you should be in the list, let me know, and I'll add :-)
- Keerthana Gurushankar, Math (now a PhD studentat CMU SCS)
- Kalee Rozylowicz (MSE; now a PhD student at Stanford)
- Shriti Priya (MS ECE; now at IBM TJ Watson Research)
- Zexi Liu (ECE; now a PhD student at CMU ECE)
- Tarana Laroia (ECE; now a PhD student at CMU ECE)
- Lisha Zhang (BME; now a BioE PhD Student at UCLA)
- Ritabrata Ray (now a PhD student at ECE, CMU)
- Shquetta Johnson (ECE; now at Intel)
- Daniel Sneider (ECE/BME)
- Xinhe Zhang (BS, MS ECE; now a PhD student at Harvard)
- Sara Caldas-Martinez (now a PhD student in Biology, CMU)
- Rachel (Rui) Sun (MS; now at Takeda Pharma)
- Shilpa George (MS; now at PhD student at CMU)
- Susan Cheng (UG, PostBac; now a PhD student at NUS)
- Ritesh Kumar (MS BME; now at Neuralink)
- Arnelle Etienne (UG; now the Director of Accessibility at Precision Neuroscopics, Inc.)
The epithet “students” is unfair to all of the above who have taught me a lot during our collaborations.
Lab visitors
- Chris Blake (UToronto; June 2016)
- Pooja Vyavahare (IITB; Summer 2014)
- Karthik Ganesan (Stanford; March 2013)
- Tongxin Li (CUHK; Summer 2014)
Research support
- NSF-ECCS-1343324 (through NSF EARS program)
- NSF-CCF-1350314 (through NSF CAREER award)
- SRC SONIC Center
- NSF-CNS-1702694 (through NSF WiFiUS program)
- Seed grants from NSF CSoI (the NSF Center for Science of Information)
- NSF CSoI affiliate
- Google Faculty Research Award
- CMU BrainHUB Award
- CMU CIT Incubation Award
Other
My favorite picture.