Space-Time Shapelets for Action
Recognition
People:
Dhruv Batra, Tsuhan Chen, Rahul SukthankarKeywords:
Action Recognition, Mid-level spatio-temporal features, bag-of-words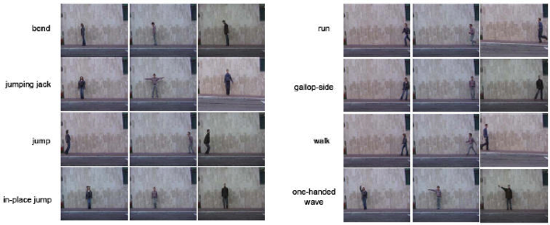
Figure
1: Weizmann Dataset: The rows represent different
actions, while the columns show different people
performing those actions.
Abstract
Recent works in action recognition have begun to treat actions as space-time volumes. This allows actions to be converted into 3-D shapes, thus converting the problem into that of volumetric matching. However, the special nature of the temporal dimension and the lack of intuitive volumetric features makes the problem both challenging and interesting. In a data-driven and bottom-up approach, we propose a dictionary of mid-level features called Space-Time Shapelets.1 This dictionary tries to characterize the space of local space-time shapes, or equivalently local motion patterns formed by the actions. Representing an action as a bag of these space-time patterns allows us to reduce the combinatorial space of these volumes, become robust to partial occlusions and errors in extracting spatial support. The proposed method is computationally efficient and achieves competitive results on a standard dataset.
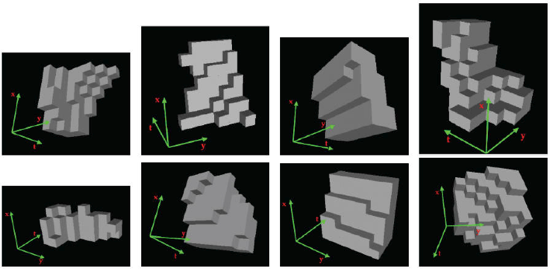
Figure
2: Space-Time Shapelets: Shown are the data-points
closest to a few cluster centers (pseudo-medians),
created from 7x7x7 volumes. The indicated temporal
dimension makes it easier to visualize motion.
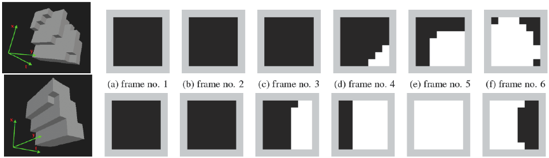
Figure
3: Unrolling volumes: Each row depicts a shapelet as
a volume, and then x-y time slices, or frames that
make up these volumes. In the frames, white
represents object pixels, black represents
background, and gray pixels exist for illustration
purposes to provide contrast.
Publication:
(Oral) Dhruv Batra, Tsuhan Chen, and Rahul Sukthankar. Space-Time Shapelets for Action Recognition. Workshop on Motion and Video Computing 2008 (WMVC '08), IEEE Winter Vision Meetings.
[ pdf ]